Everyone you talk to these days is somewhere in the AI adoption process. In fact, IBM found that almost 80% of businesses have adopted AI or have a plan for it. The enterprise AI market is estimated to grow at a CAGR of 44.1% from 2023 to 2032 (Precedence Research, 2022).
But diving headfirst into AI development isn't always smooth sailing. Some companies waste millions on a failed AI project. The result is unused infrastructure and useless technologies they try to make work.
Follow this 5-stage comprehensive guide for enterprise IT leaders to ensure your AI project is set for success.
Stage 1: Avoid AI project failure with strategic alignment
Do not just jump into an AI project because everyone else seems to be doing it. Before you invest any hard resources like time and money, make sure to align the project with the company’s strategic goals. You can talk to AI experts, vendors, or peers who are using AI for identifying specific use-cases or pain points AI can address.
Challenges/Risks
Misalignment of AI initiatives with core business objectives can lead to wasted resources and lack of executive support.
How to mitigate the risk
Ensure the AI projects directly support and enhance the company’s strategic goals, and the expected ROI is realistic.
Stage 2: Hire a top-tier data scientist for $900,000/year
Build a multi-disciplinary AI team, by hiring data scientists, AI researchers, data engineers, IT personnel and domain experts. You can also educate existing personnel with ongoing training, but it will take longer than hiring a skilled professional, so balance different talent acquisition options.
Challenges/Risks
Skilled AI talent is in high demand, so expect hiring challenges or to pay top dollar.
How to mitigate the risk
Make sure you have someone on your team capable of distinguishing real talents to avoid wasting money on wrong hires. You can also collaborate with external AI experts or agencies.
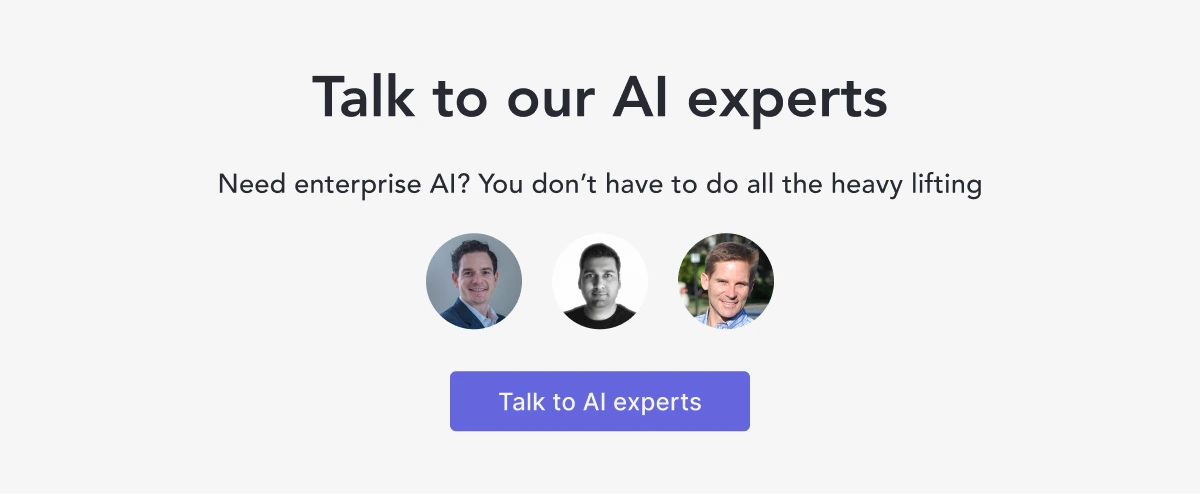
Stage 3: Clean, label, and prepare petabytes of data
Audit the current data infrastructure. Inconsistent or low-quality data can severely undermine AI models. Raw data is not typically usable. Have your new AI team go over the quality of the data and see if they can treat the data as AI-ready.
Challenges/Risks
A famous saying in the AI community: Garbage in, garbage out. Data in enterprises are typically stored in different formats, in disparate datasets, and sometimes even on paper. S&P Global survey revealed that data management was one of the top challenges in building enterprise AI.
AI initiatives are "forcing a complete rethink of how data is stored, managed and processed," said Nick Patience, senior research analyst at S&P Global Market Intelligence.
How to mitigate the risk
Have the budget ready to support developing or upgrading to a robust data warehouse or data lake solution. If the current data turns out to be unusable for the business objective and AI use case, use the budget to acquire external data, yet it may be costly.
Don’t forget to implement tools and procedures for ongoing data collection, cleaning, and validation. Ensuring scalability will save you headaches and skyrocketing costs.

Stage 4: Invest in the right AI deployment infrastructure
Decide where to run your AI model. There are 3 different deployment options: cloud vs. on-premises vs. hybrid deployment based on data sensitivity and infrastructure readiness.
It is common for enterprises to process large amounts of data. This makes the on-prem deployment option the most cost effective. You can procure NVIDIA GPU chips (DGX model) for $199,000 - $400,000 a piece depending on how much your AI has to process.
Challenges/Risks
Each option comes with different cost variability and choosing the wrong option may incur millions that could have been saved with a different deployment option. Also, avoid choosing a technology that becomes obsolete quickly or doesn't integrate well with other systems. It can skyrocket the maintenance cost.
How to mitigate the risk
Opt for widely adopted, well-supported platforms. Ensure flexibility and scalability in the technology stack to adapt to advancements. Choose an infrastructure that can expand as required. Utilizing cloud platforms allows rapid scaling.
Stage 5 will cover the importance of scalability further. Use this full comparison chart for cloud, on-prem, and hybrid deployment options for better decision making.
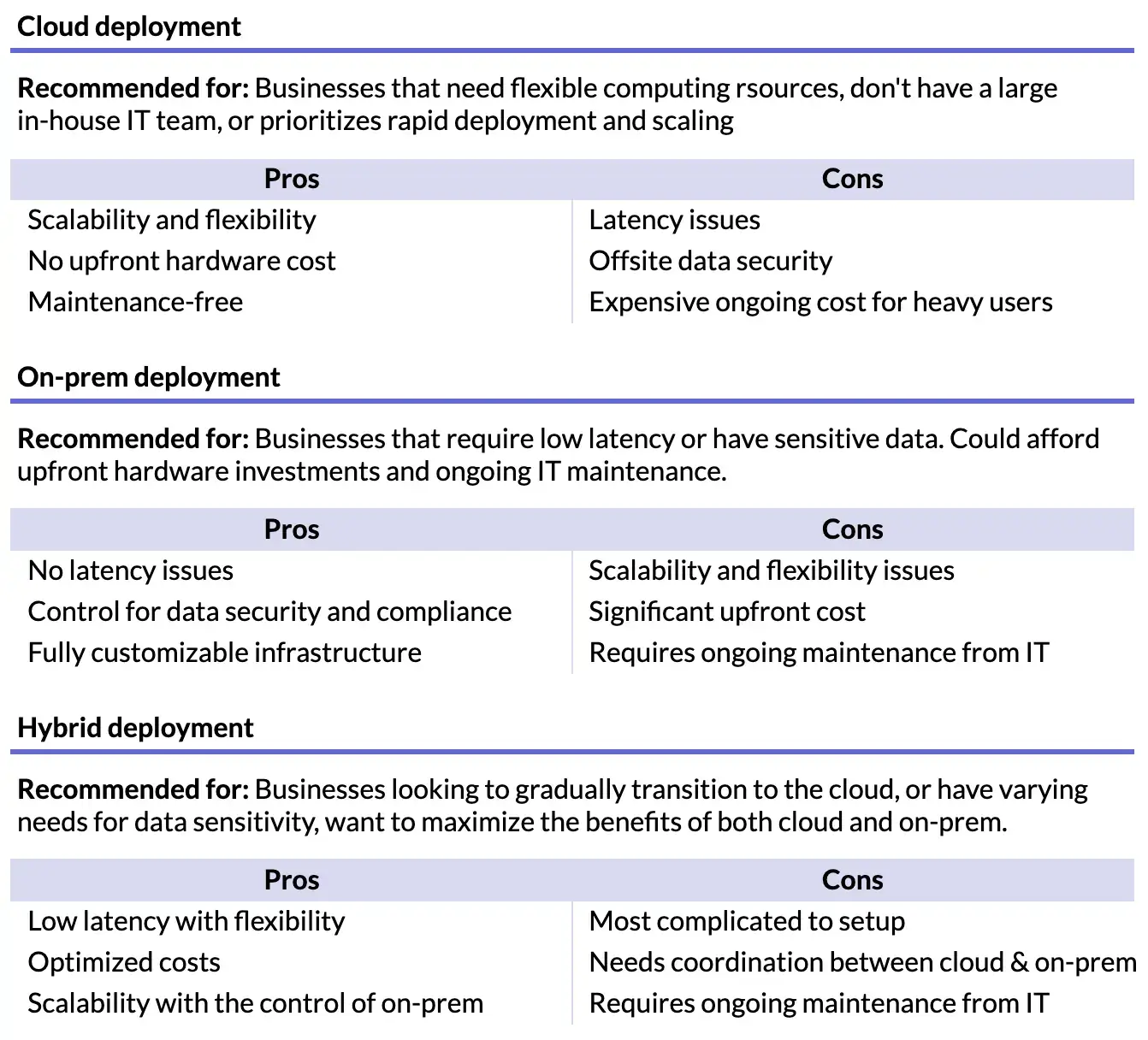
Stage 5: Ensure scalability at all cost
Scalability means that as the data input grows, the model can still process it efficiently and provide accurate predictions or analysis without significant performance degradation.
Challenges/Risks
A non-scalable AI model will slow down predictions or analysis (performance bottlenecks). Not providing timely real-time insights or predictions can cause operational disruptions, and even operational failures if other critical systems or solutions rely on AI-produced data.
Non-scalable AI models mean frequent manual intervention and infrastructure upgrades, skyrocketing maintenance costs.
Costs of a non-scalable AI model
- Financial Costs: Manual interventions, frequent upgrades, and the need to build or buy additional resources can lead to significant expenses.
- Reputational Costs: Delays, downtimes, or incorrect results due to performance issues can damage the company's reputation.
- Operational Costs: Continuous monitoring and intervention required to keep a non-scalable system running can tie up human resources.
- Opportunity Costs: The organization might miss out on new business opportunities or fail to recognize emerging patterns in data.
How to mitigate the risk
If you’ve successfully implemented stages 3 and 4, you should have a scalable data management solution and infrastructure to support AI scalability. Regularly check in with your AI team:
- Distributed Systems: Implement distributed computing solutions that can process large datasets by distributing the workload across multiple machines or nodes.
- Model Efficiency: Optimize the AI model for performance. Some deep learning models can be quite large and require significant computational resources. Techniques like model pruning, quantization, and efficient neural architecture search can help.
- Batch Processing: If real-time processing isn't a requirement, processing data in batches can be more efficient than processing each instance individually.
Pro tips: Things to keep in mind
Tip 1: Start small
Even with top AI talents, AI models will have bugs, problems, and issues like all technologies. A big project that fails will result in millions of resources wasted and a complete project halt.
- Initiate small pilot projects to validate the feasibility of larger AI projects.
- Use these pilots to refine the team's approach and to get early buy-in from stakeholders.
- Set clear, modest expectations for prototypes and use failures as learning experiences.
Tip 2: Don’t forget about ethical and regulatory considerations
Understand the potential ethical implications of the AI models, especially if they affect customers or employees. Neglecting ethical considerations can lead to public relations disasters, while regulatory non-compliance can result in penalties.
- Ensure that AI deployments are compliant with regional and industry-specific regulations, especially around data privacy.
- Stay updated on AI ethics discussions and industry-specific regulations.
- Involve legal teams in AI project planning.
Tip 3: Develop a contingency plan
Anticipate potential risks, both technical (like model failures) and strategic (like misalignment with business goals). Ensure that the business can continue smoothly even if AI encounters issues with a plan for these risks.
- Conduct regular risk assessments and prevent crisis.
- When related to the critical operations, have backup AI models or systems ready to run to avoid disruptions.
Remember: You don’t have to do the heavy lifting
It’s been years since Amazon launched their first AI-powered smart store. Since then, many technology providers have developed AI to solve specific problems that enterprises have and made impactful AI use cases available to the market.
Building AI from scratch in-house takes time and resources without promising a 100% success rate. Remember that there are many ready-to-use AI solutions that can be customized and tailored to your specific enterprise environment so don’t limit your AI initiative options to building it in-house.
Anyone can evaluate options like AI experts using this complete step-by-step AI buying guide.
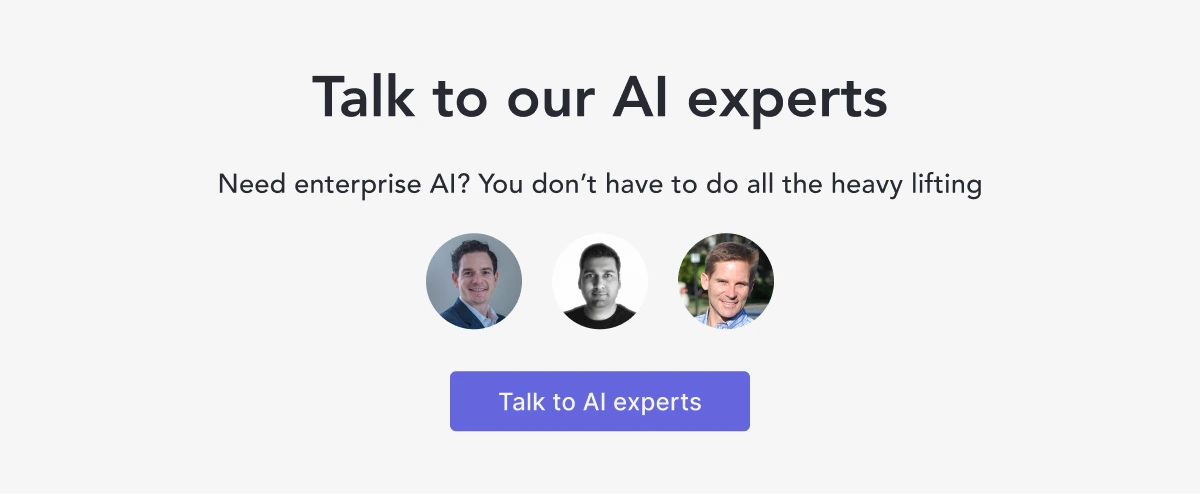